ECE PhD Candidate Nishatul Majid will present his dissertation Design of an Offline Handwriting Recognition System Tested on the Bangla and Korean Scripts on Monday, April 27.
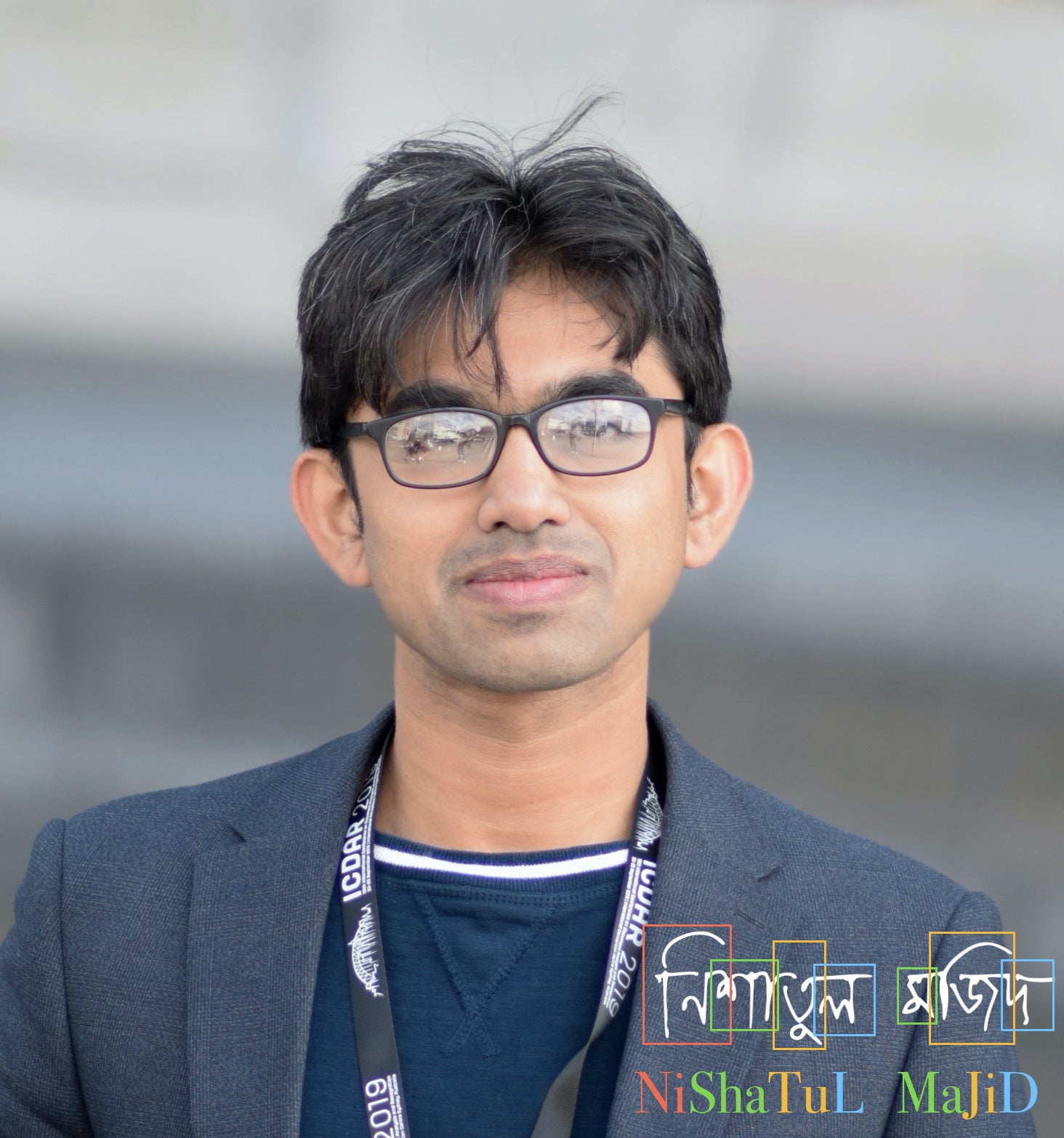
Majid’s dissertation presents a flexible and robust offline handwriting recognition system that is tested on the Bangla and Korean scripts. Offline handwriting recognition is one of the most challenging and yet to be solved problems in machine learning. While a few popular scripts (like Latin) have received a lot of attention, many other widely used scripts (like Bangla) have seen very little progress. Features such as connectedness, vowels structured as diacritics make it a challenging script to recognize. A simple and robust design for offline recognition is presented which not only works reliably but also can be used for almost any writing system. The framework has been rigorously tested for Bangla and demonstrated how it can be transformed to apply to other scripts through experiments on the Korean script whose two-dimensional arrangement of characters makes it a challenge to recognize.
The base of this design is a character spotting network which detects the location of different script elements (such as character, diacritics) from an unsegmented word image. A transcription is formed from the detected classes based on their corresponding location information. This is the first reported lexicon-free offline recognition system with a Character Recognition Accuracy (CRA) of 94.8% for Bangla. This is also one of the most flexible architecture ever presented. Recognition of Korean was achieved with a 91.2% CRA. Also, a powerful technique of autonomous tagging was developed which can drastically reduce the effort of preparing a dataset for any script. The combination of the character spotting method and the autonomous tagging brings the entire offline recognition problem very close to a singular solution.
Additionally, a database named the Boise State Bangla Handwriting Dataset was developed. This is one of the richest offline datasets currently available for Bangla and this has been made publicly accessible to accelerate the research progress. Many other tools were developed and experiments were conducted to more rigorously validate this framework by evaluating the method against external datasets. Offline handwriting recognition is an extremely promising technology and the outcome of this research moves the field significantly ahead.
Nishatul Majid is in his final year of study as a doctoral candidate in the Electrical and Computer Engineering Department at Boise State University. He completed his bachelor’s and master’s degrees in applied physics, electronics and communication Engineering at the University of Dhaka in Bangladesh. His research interests lie at the intersection of image processing and machine learning. Majid is supported in his doctoral research by ECE professor and advisor Dr. Elisa Barney and his supervisory committee, Drs. Rafla and Smith.