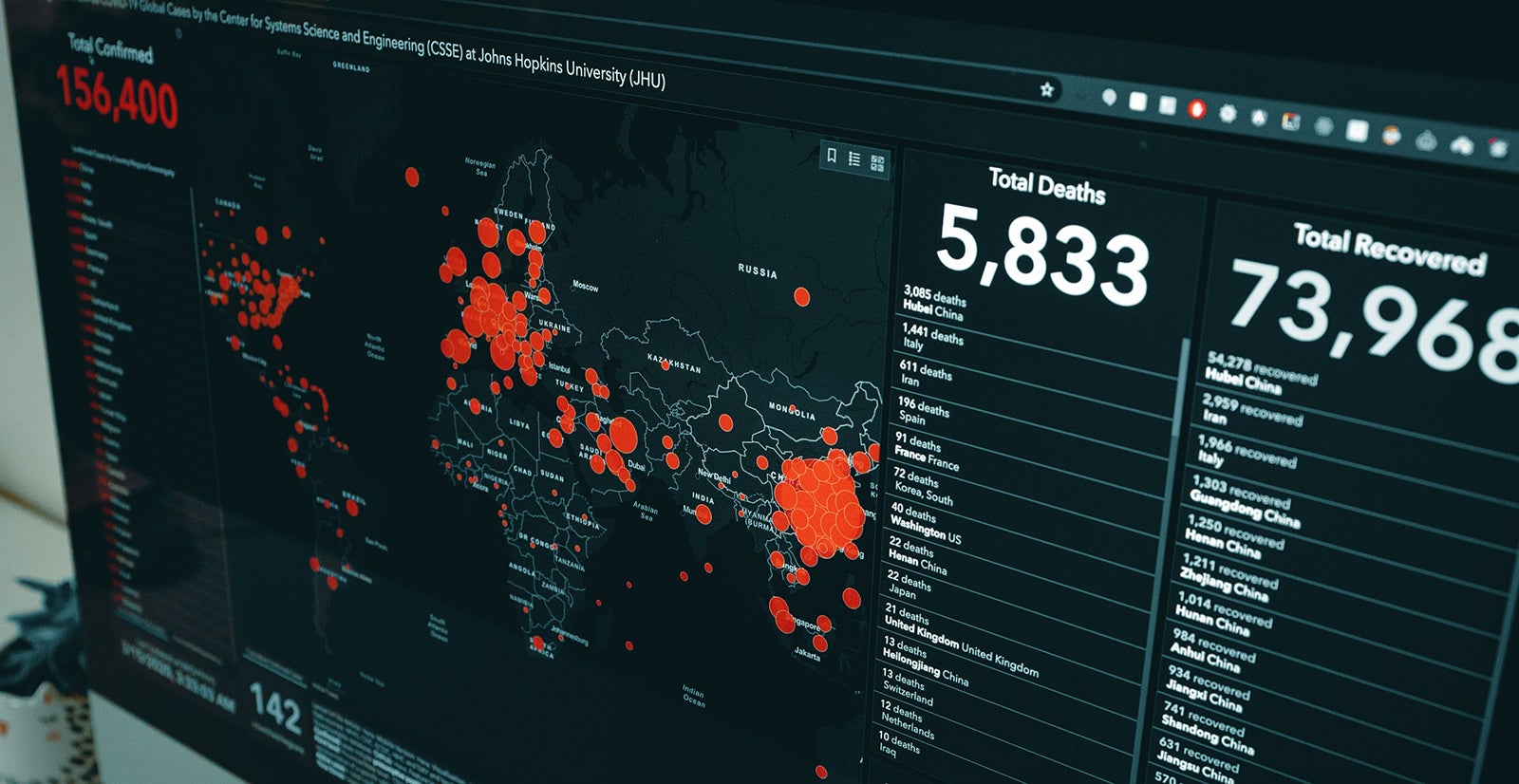
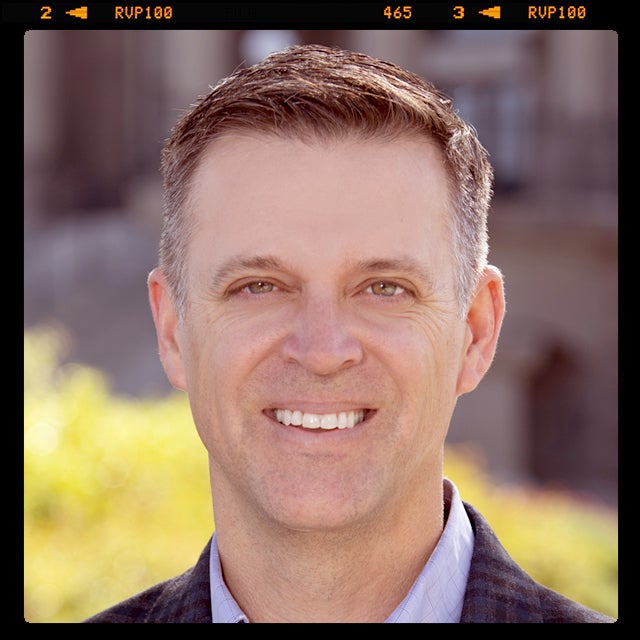
Greg Hill earned his PhD from Texas A&M University in 2006. Hill has extensive research and survey experience in the academic, public, and private sectors, including producing and analyzing data for many of Boise State’s public policy surveys. His interests center on public management, administration and policy theory and immigration policy. He is an avid baseball fan and has twice been invited to present his baseball-related research at the Cooperstown Symposium on Baseball and American Culture at the National Baseball Hall of Fame.
These days, from almost all information media sources, we are inundated with articles, data, graphs, and even memes on COVID-19. As we sift through this information, we may ask,
Why are all of these models so different?!? How is it that so many mathematical models of the COVID-19 outbreak can vary so widely? Which ones can we trust? Which ones can we understand?
This article by Koerth, Bronner, and Mithani at the fivethirtyeight.com blog does an excellent job in describing this variation. One relevant quote: “Over the next few months, you are going to see many different predictions about COVID-19 outcomes. They won’t all agree. But just because they’re based on assumptions doesn’t mean they’re worthless. All models are wrong, it’s striving to make them less wrong and useful in the moment.”
Here are some things to consider while you are trying to absorb these models:
- Look for transparency – It should be very easy to find a technical report somewhere on the webpage. In the technical report you should be able to find a list of variables, where the data come from, how up to date the data are, and what assumptions the model builders are using. If you cannot find a technical report attached, be wary of the reliability and validity of the model.
- Know the assumptions – Because models are predictions, they are not using actual data in the projections. They are making assumptions with the data that exists to get to those projections. Understanding what these assumptions are is especially useful when you are comparing reports. For example, some modelers prefer to use best case scenarios, others worst case. Sometimes they are using logarithmic equations, sometimes they are using linear equations. Knowing the nature of the models can help you interpret the results.
- Look at the sources of data and who made the model – Not all models (or modelers) are created equal, and not all are made for the same purpose. Looking at where the data comes from (is it from a reputable organization, is it from a think tank or political party?) will help you understand the purpose of the projections. Who is making the model? Who is the audience? Being able to answer these questions will shed light on how to interpret the results.
Data-driven projection models are important to decision making processes, however, you as a consumer of these models need to be “in the data.” Hopefully these three considerations will help you as you sift through the many projections out there. And don’t forget to read Silver’s article!
Note: This article is part of The Blue Review’s Coronavirus Conversations, a special series on the 2020 COVID-19 pandemic.