Dr. Li’s group utilizes first-principles density functional theory-based methods to couple with molecular dynamics, phase-field modeling, data science, AI/machine learning and experiments. The research spans from materials growth to property screening, from device simulation to materials informatics. Dr. Li’s group widely collaborates with national labs, industry and other universities, including Naval Research Laboratory (NRL), National Institute of Standards and Technology (NIST), Idaho National Lab (INL), Center for Advanced Energy Studies (CAES), Boeing, Brewer Science Inc. Dr. Li has received funding from DOE, ONR, NSF, NASA and NIST.
In This Section:
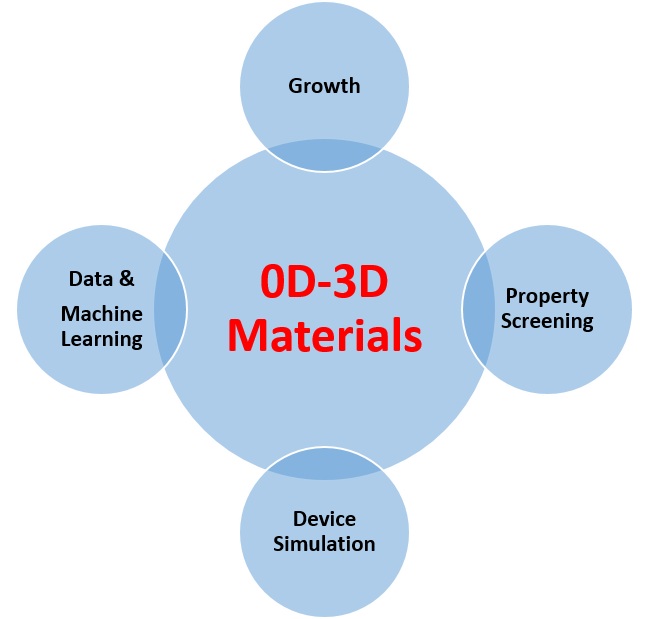